Understanding trends and forecasting future opportunities and shifts in customer behaviors as well as production patterns are critical in the eCommerce fulfillment space. This is especially important for brands looking to scale. The opportunity to fulfill a greater number of customer orders is immense.
But at the same time, the risk involved, whether that’s unsold stock or warehousing costs for products that move slowly, scales just as much. Two analytics methodologies - descriptive analytics and predictive analytics can empower businesses to gain insight into the past and plan for the future to mitigate the risks involved with scaling.
What is Descriptive Analytics?
Descriptive analytics relies on historical data aggregated over time to describe what happened in the past in detail. For instance, keyword data mining from customer reviews could tell that a particular product variant performed exceptionally well, met customer needs, and was seen as excellent value for money.
On the flip side, a low average star rating for a particular product on an eCommerce platform could tell that it has quality control issues or otherwise failed to deliver the experience expected. Descriptive data essentially describes what happened and tells how and why.
Based on the big data from the descriptive analysis, one can predict future outcomes and craft a better way to handle specific situations.
Descriptive analysis can help identify trends in customer behaviors and help predict where there is likely to be a demand.
What is Predictive Analytics?
On the other hand, predictive analytics helps one make educated predictions about what could happen in the future. Predictive analytics also leverages past data, but instead of using it to describe the how and why of what’s already happened, predictive analytics helps businesses forecast future possibilities.
For instance, historical information on seasonal shifts in demand for a product like a winter clothing could help an eCommerce player predict the expected demand for next year and the volume of winter clothes in stock. Predictive analytics enables businesses to adopt a proactive approach to decision-making, planning, and implementing decisions ahead of time.
Many eCommerce players face a challenge: leverage Legacy WMS (warehouse management systems). These older software suites tend to focus on descriptive analytics and lack predictive capabilities. While Legacy solutions are of great help when it comes to post-mortems on prior situations (e.g., “why did we see a sudden decline for demand for product line X last year leading to overstocking,”), they’re less effective when it comes to planning for the future.
In this context, newer OMS (order management systems) with real-time tracking capabilities offer significant advantages thanks to predictive analytics modeling.
How exactly can predictive analytics help eCommerce businesses achieve their goals and scale? Let’s take a look now at specific use cases.
Use Cases and Scenarios for Ecommerce Predictive Analytics
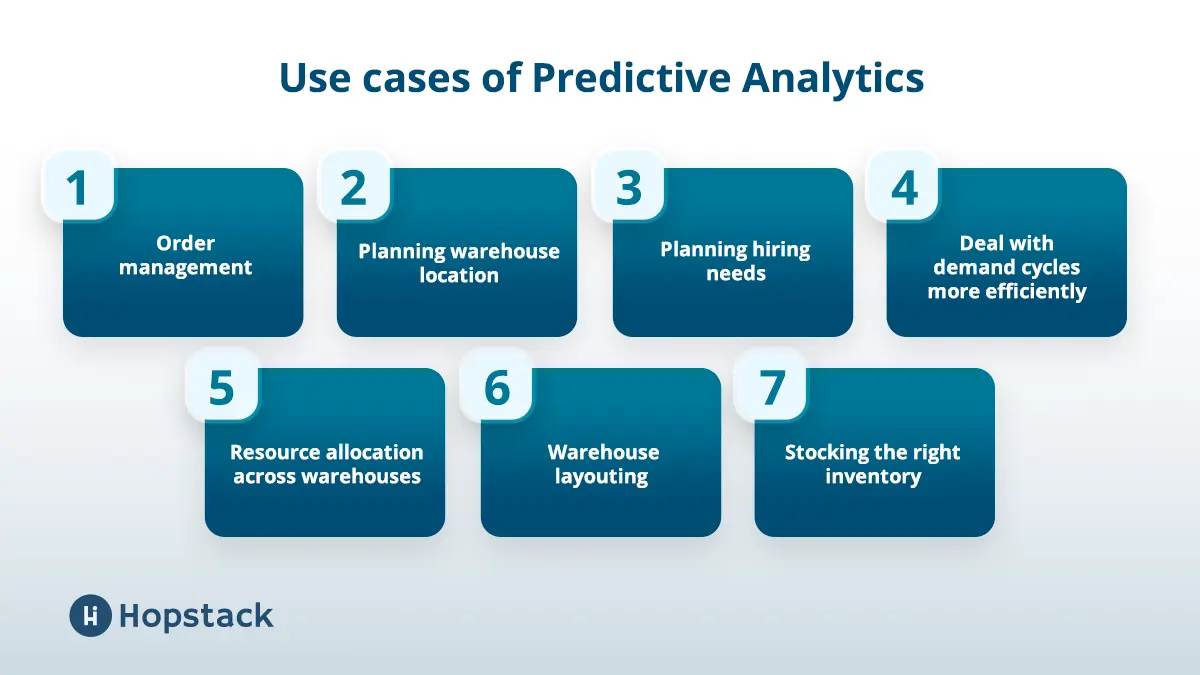
Order management
Every step of the fulfillment process has areas of opportunity where predictive analytics-backed decisions can help save on costs and time. Modern warehousing needs a powerful WMS that can leverage historical data and can create predictive models to optimize order batching, pick/pack/ship operations, and enable smart warehousing associates to prioritize the orders depending on order source, customer, geography etc. This helps warehouse managers save time and boost warehouse throughput by preparing for the situation in advance.
Helps in planning the warehouse location
For most brands, customers aren’t evenly spread across a given region. There are often clusters of customers of a demographic in certain areas and other areas with few or even zero potential customers.
Further, there is no guarantee that suppliers are located close to customer clusters. This makes positioning warehouses in the right locations critical for timely order fulfillment. Mining data on customer locations can help identify areas with the greatest customer density (e.g., well-off urban areas if one is an online electronics retailer).
By evaluating particular data about existing customers (their income levels, addresses, age range, etc.), predictive analytics solutions can help identify the geographic areas where potential customers are most likely to live. Using this information, one can decide on warehouse locations that ensure that the maximum number of potential customers have their orders fulfilled on priority from close-by warehouses.
Helps in planning hiring needs
When scaling up order fulfillment capabilities, one needs more workers to deal with transport, handling stock in warehouses, and a range of other functions. Employees can be a significant fixed cost center, however. For example, Amazon, to face the holiday season demand surge, hired about 150,000 temporary workers in 2021. They arrived at this number by analyzing historical data on seasonal demand and predicting the same for 2021.
Hire too many staff and risk underutilizing them while cutting heavily into margins. Hire too few and may face capacity issues and fulfillment delays. Predictive analytics solutions can help model staff member capabilities against needs to answer questions like “how many warehouse staff does one need to ensure we’re smoothly handling 2500 units of product X every week without fulfillment delays?”
Deal with demand cycles more efficiently
Many products have cyclical patterns of demand. This is especially true with seasonal goods. For instance, no one needs a winter jacket in the middle of summer. What does this mean for eCommerce players? Having a sound understanding of demand cycles can help plan out everything from stocking inventory to advertising spending on specific products to ensure that supply aligns with changes in demand.
Predictive analytics solutions can evaluate historical demand cycles to help pinpoint peaks and troughs in order and ensure that the business is fulfilling customer needs without overextending spending. Powerful analytics platforms can make such recommendations and forecasts way ahead of time for businesses to prepare ahead of time.
Resource allocation across warehouses
This point parallels what the article discusses earlier about warehouse location. After examining historical data on customer density across geographical locations, businesses can identify which warehouses are “priority” in terms of the volume of products they handle and where to allocate resources like transport vehicles and warehouse staff.
Warehouse Layouting
Predictive analytics can help immensely in providing insights for warehouse layout and how SKUs are to be placed across the IoT warehouse area. Past data on inventory velocity, picking operations, replenishment rate, etc. help in determining the optimal area for each product and can also help in streamlining various inbound and outbound processes.
A powerful data-driven WMS should be able provide layouting recommendations not just based on past data but also the future trends and demand/supply cycles it forecasts.
Helps with stocking the right inventory
Inventory optimization is perhaps the greatest challenge for scaling up an eCommerce operation: buying up inventory is a high cost, and unsold stock due to changes in demand could lead to immense loss. Predictive analytics solutions can help mitigate risks in inventory management.
By evaluating historical data on customer buying patterns and demand shifts, predictive solutions can help determine the approximate quantity of inventory it makes sense to stock to ensure timely order fulfillment while mitigating the risk of ending up with unsold units on hand. Overstocking can be extremely detrimental to a company’s operations and finances. In fact, the carrying costs can make up for about 25-30% of the total inventory cost.
This can help in several ways:
Predict stock out situations
Predictive analytics will help ensure that the businesses have enough inventory in stock to fulfill customer demand well within the fulfillment timeframes that buyers expect. This’ll prevent situations when the inventory’s out of stock and cannot fulfill customer orders on time and avoid disruption due to shipping delays.
Predict supplier disruptions
Supplier disruptions can have just as much of an impact on the end customer experience as an out-of-stock situation at the putaway warehouse. The suppliers deal with several externalities that can periodically limit their ability to fulfill orders. Predictive analytics can help understand market situations in which supplier disruptions are more likely and prepare by stocking up.
Predict revenue figures
How much does one likely make from a particular product lineup next quarter? What about overall revenue expectations? Having a clear picture of these factors is important in planning out future decisions - how much to invest next year in digital ads, for instance, or whether or not to move into a new product category. Predictive analytics can look at prior revenue performance and, factoring in growth expectations, give a reasonably clear picture of the revenue that one is likely to make in the near and medium future.
In order to maximize the efficiency of predictive analytics, businesses should consider leveraging Robotic Process Automation (RPA) to automate mundane tasks and reduce errors. RPA can help streamline customer service, finance and accounting, human resources, telecom, retail, healthcare, IT operations, manufacturing, data validation, and reporting processes. By combining predictive analytics with RPA, eCommerce businesses can gain a competitive edge and ensure that their operations are running smoothly and efficiently.
Conclusions on Descriptive Analytics and Predictive Analytics for E-commerce Fulfillment
Forewarned is forearmed: understanding potential future trends and taking action accordingly is a significant asset for eCommerce businesses. Predictive analysis helps one to do just this. By mining a wide range of historical data, predictive analytics tools can help extrapolate possibilities - in terms of growth, changes in demand, competition, and so much more. If one is planning to scale their eCommerce business, this knowledge is all the more important. Making decisions ahead of time can help mitigate the risk environmental changes while empowering to make decisions that maximize profit and bolster resilience to changes in the market.
Schedule a demo with Hopstack to learn more about how automated warehouse platform and execution platform can analyze historical data and help provide business recommendations on order fulfillment, inventory optimization, outbound operations, and warehouse resource allocation.